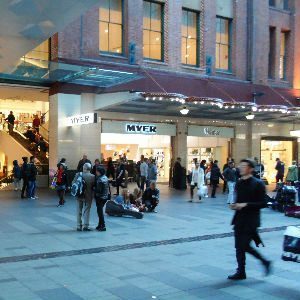
Retail In-Store Analytics
Find and compare in-store analytics companies and solutions. Measure shopper behaviors and increase store conversions.
When people hear “retail analytics”, they often immediately jump to intelligent and connected marketing. While this is a good portion of the puzzle In-store analytics can help drive retail value in a variety of ways.
Advanced algorithms have been developed to take in anything from video to images to audio and presence sensors and attempt to break it down into discrete chunks of meaningful information for a store. Once categories of information are established, trends can be established by tracking progress over time, ultimately allowing a business to alter their techniques in order to save money, manage their building and equipment more efficiently and engage customers and every step of their journey both when they first enter the store, and continuing well after when they have returned home.
The following Channel Guide will help you:
- Determine the best customer analysis tools and the technology behind brick and mortar traffic smart sensors, video analytics, etc
- Give overview of Bluetooth beacon and Wifi tracking technologies and their security concerns
- List resources on forward looking technology like predictive analytics and big data analysis for physical retail stores.
11/01/2019
Solution Providers
Companies with physical retail analytics solutions.
Proximity / Beacon / Location
Foot Traffic / People Counting
Shelf / Shopper Focused
Additional
Retail analytics video analysis
Location Focused:
Beacon Payment Focused
Investments & Acquisitions
Investments
- RetailNext raises $125M (4/2015)
- Placemeter raises $6M Series A (9/2014)
Acquisitions
- ACTITO has acquired SmartFocus (6/2019)
- Placemeter acquired by Netgear (12/2016)
- SmartFocus Acquires TagPoints and Content Savvy (9/2014)
For a more complete list of acquisitions and investment activity download our Smart Buildings Report.
Additional resources
Resources:
- Article: Retail Touchpoints - In-Store Insights Help Turn Browsers Into Buyers
- Article: Forbes - E-Commerce Style Big Data Analytics Meet Brick And Mortar Retailers
- Article: NYTimes - How Companies Learn Your Secrets
- Article: RetailNext - Improved Wi-Fi chip sets will benefit in-store analytics
- Article: Revolution Wi-Fi - 5 Retail Trends Driving Wi-Fi
- Research: McKinsey Global Institute - Big data: The next frontier for innovation, competition, and productivity
- - CQuotient - Impact of “Big Data” on Retail: The McKinsey View (Part 1 of 2)
- Research: Babson - Realizing the Potential of Retail Analytics Plenty of Food for those with the Appetite (pdf)
- Research: PSFK - Future of Retail
- Interview: Oreilly Radar - Google Analytics for the Real World: A Conversation with Sharon Biggar of Path Intelligence
Privacy:
- Mobile Location Analytics Code of Conduct (PDF)
- Article: FastCo - Here’s What Brick-and-mortar Stores See When They Track You
- Article: Bloomberg - Big Brother Watches as Stores Seek More Data: Retail
- Article: WSJ - Tracking Companies Agree to Notify Shoppers, But Retailers Demur
Product Spotlights
A closer look at some of the products live in the marketplace.
Scanalytics SoleSensor
Physical Consumer Analytics
With a name like Scanalytics, you might think the company’s presence-tracking platform would rely on some kind of proximity beacon to detect people via their smartphones — but in fact it’s much simpler. The SoleSensor is a smart floor mat that can hide under the carpeting, quietly and anonymously tallying footfalls to provide a snapshot of how many people pass by, how long they linger, and where they head next.
A single mat can be placed at a critical spot, or a series can be daisy-chained together to cover large areas or entire rooms. The embedded pressure sensors are more accurate than infrared beams for tracking who comes and goes, and can extrapolate direction, speed, and other data for each individual who crosses them.
For retailers, customer monitoring is all about figuring out how to get patrons to pay attention to products. That means analyzing the movement patterns of customers as they enter or leave and strategically placing displays where they’ll draw the most attention — and that’s exactly what SoleSensor is designed to do.
Carpet a store with the sensor mats, and managers can answer questions like: Which direction do customers head as they enter? How long do they spend in front of the interactive demo in aisle six? How long are others willing wait for a turn at the demo before moving on? They can also link customer behavior to other smart devices, for instance by having a screen display additional information whenever a customer stands in front of it for a specific length of time.
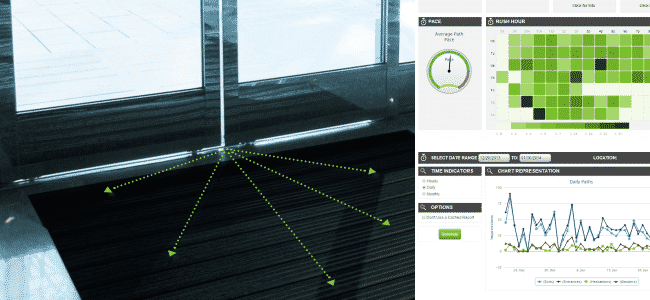
While retail is among the most obvious use cases, SoleSensor has applications for just about any physical space humans occupy, from the smart home (automatically turn appliances on/off) to healthcare (monitoring patients) to large event spaces and conference venues (measure crowd size and movement).
Visit ScanalyticsInc.com to view case studies, or request access to the company’s SDK and develop new products and applications based on SoleSensor technology.
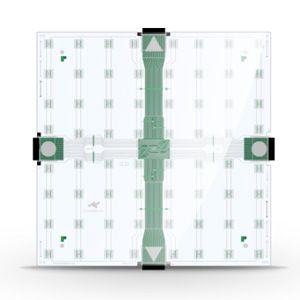
Density Counter
Foot Traffic Counter
Density is, as the company’s website puts it so simply, a people counter. Like other startups that analyze foot traffic, Density is designed to let retailers, coffeeshops, co-working spaces and other places collect better data about how many customers pass through their doors.
In conversations at online forums, Density’s founders are candid about their belief that hardware sales are becoming a tough game. That’s why the company’s own device is both simple and free. It’s essentially just a pair of infrared sensors, with parallel beams crossing a doorway so it can count the people passing through and know whether each one is coming or going. Getting and installing the hardware costs nothing; Density makes its money through subscriptions to its data-analysis service.
Counting customer visits is plenty useful for retailers and other venues, but as Density’s name implies, there’s a particular value in having an exact count of the number of people in a location at any given time. Grocery stores could use it to anticipate a rush at the checkout counters, and have more cashiers available before the lines build up; bars and dance clubs could use it to more precisely meter the flow of people through the door line.
The value of the information can also be passed on to customers by making the data available online or in an app. Imagine being able to check how crowded the local coffeeshops are, so you can pick a quiet place to have a conversation; or knowing before you leave home whether there’s an open desk at your local co-working space.
Infrared sensors aren’t the only way to count people, as the diversity among Density’s competitors proves. Technologies in this area range from Wi-Fi beacons that detect customers by pinging their smartphones, to pressure-sensitive floormats that count customers by their footsteps, to camera (or camera-like) systems that count people with machine-vision algorithms. And of course there’s the old “break-beam” sensor, which has been in use for decades, but tends to be neither networked nor capable of telling whether each person is headed in or out of a building.
Density is promoted as combining the best features of all these options: cheap and simple hardware, accurate real-time data, and completely anonymous. While competitors like Placemeter have to go out of their way to assure the public that their sensors are not really cameras and that they’re not using face recognition or other ways of identifying individuals, Density’s data is so low-fi that it undercuts most privacy concerns while still delivering useful insights.
Learn more at Density.io.
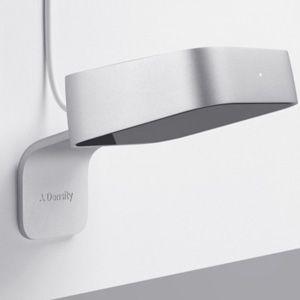
Zensors: Crowdsourced Visual Sensing
The Internet of Things is practically littered with sensors—small, purpose-built devices that are exceptionally good at detecting a particular aspect of their environment, like temperature or motion. But turning IoT sensor data into useful information (How cold is it outside? How fast am I going?) requires some kind of program that knows, or can be configured to know, what the data means and how it should be interpreted.
Wouldn’t it be nice if instead you could just, y’know, ask a question?
That’s the idea behind Zensors, a product originally created by computer science grad students and professors at Carnegie Mellon University.
Zensors repurposes unused smartphones as camera-equipped visual sensors, and uses a combination of crowdsourcing and machine learning to answer natural-language questions about what the camera sees. The work was presented at the CHI ‘15conference on human-computer interaction in Seoul earlier this month.
In a live demonstration, PhD student Gierad Laput showed how the Zensors app lets users mark an area of interest in a camera’s field of view by drawing a circle with their fingertip, then type a question in plain English about what’s happening in that part of the image. You might point your camera out the window and ask “How many cars are in the parking lot?” or “Is it snowing?” Or you might point it at a room to ask things like “Where’s the dog?” or “How large is the pile of dishes on the kitchen counter?”
The CMU team knew that answering any one of these questions with a specially-built machine vision application could cost thousands of dollars. Instead, they turned to crowdsourcing through Amazon’s Mechanical Turk platform, which offers small payments to human workers who complete simple on-screen tasks.
Humans have the advantage of being able to quickly understand the question being asked and make a quick visual assessment. With reasonably frequent camera readings, a week of Mechanical Turk image assessments might cost only a few dollars. At the same time, the Zensors platform learns from the human evaluations, and can take over as soon as its own accuracy is up to snuff. Then it’s just a matter of having humans check in occasionally to make sure the algorithm remains accurate over time and adapts to changing conditions (if you teach it to count cars in a parking lot during nice weather, the first snowfall may throw off the data).
A phone or wireless camera running the Zensors app can answer multiple questions about a single image, and privacy-conscious users can use blurs and other filters to obscure parts of the image that are sent off to the crowd. The platform also includes a rules engine, so the answer to each question you ask it can trigger text or email alerts or prompt behaviors in other apps and connected devices.
Of course, it’s not a perfect system. “Some sensors are just not going to work,” Laput said in the CHI presentation. “Subjective questions tend to yield very poor accuracy.” Teaching a computer to recognize an orderly line at a cashier’s station, for instance, is a lot harder than teaching it to count the number of people waiting.
There are plenty of uses for visual sensors, from home security and proximity/presence detection to more sophisticated machine vision applications, and there are plenty of IoT products offering these capabilities to varying extent. If Zensors can be commercialized, it would offer a simple and user-friendly alternative that reuses hardware—old phones—which many consumers already have lying around the house. To learn more, read through the full research paper or have a look at the video below.